The Role of AI in Data Analytics: Transforming Data into Decisions
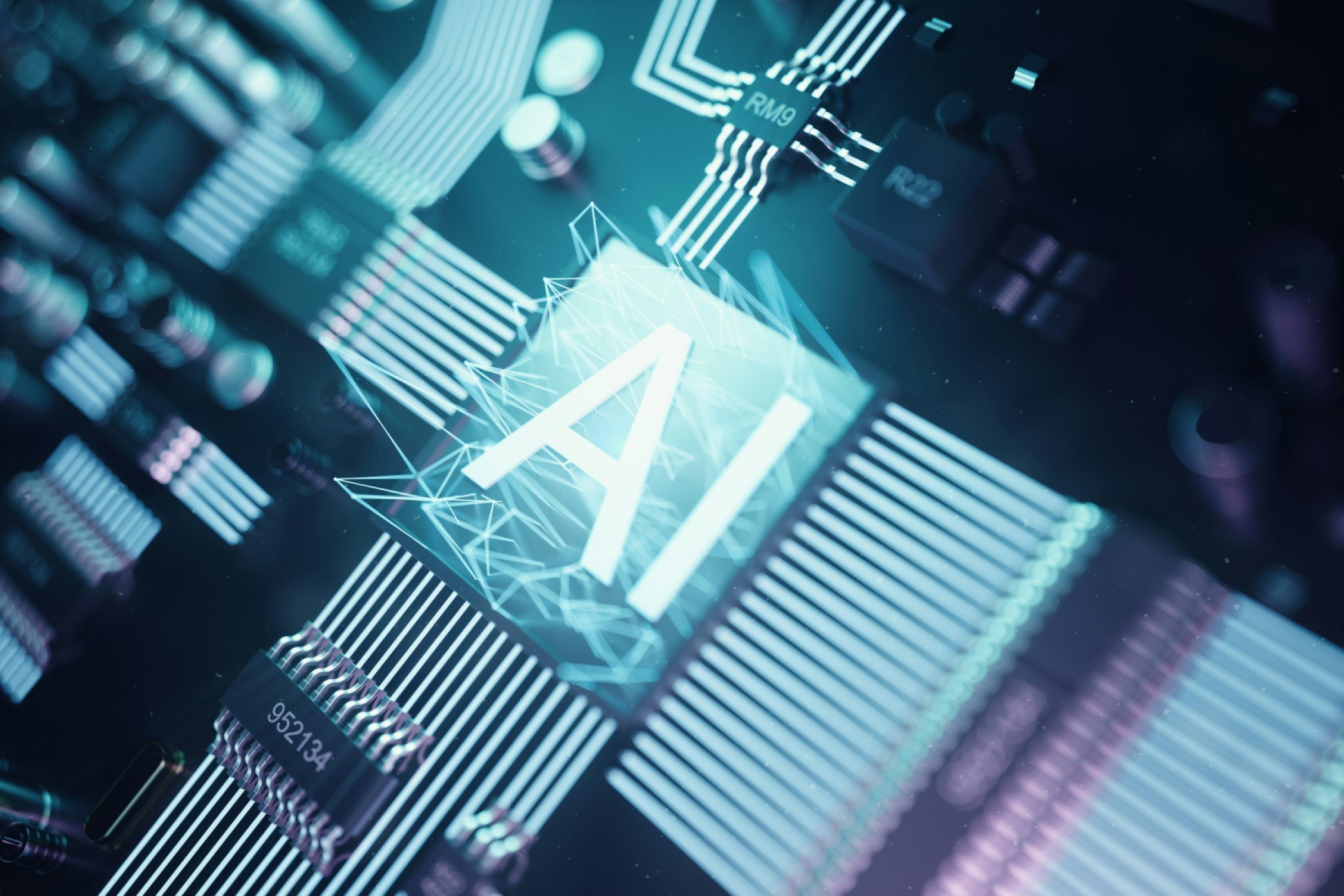
In the rapidly evolving digital landscape, the fusion of Artificial Intelligence (AI) and data analytics has become pivotal in transforming raw data into strategic decisions. This synergy is enhancing the capabilities of data analysts and redefining how organizations operate, innovate, and compete. With its ability to learn from data, predict outcomes, and automate processes, AI plays a crucial role in unlocking the full potential of data analytics. This article explores the integration of AI in data analytics, highlighting its impact on automating processes, predicting trends, and enhancing decision-making.
Automating Analytical Processes
One of AI’s most immediate impacts on data analytics is automating routine and complex tasks. Traditional data analysis requires significant human effort to collect, clean, and prepare data before any analysis can begin. AI algorithms, particularly those based on Machine Learning (ML), can automate these processes, dramatically reducing the time and resources required.
Machine Learning Models
ML models can be trained to recognize patterns and anomalies in data, automating the process of data cleaning and preparation. For example, without human intervention, an AI system can learn to identify and correct inconsistencies in datasets, such as missing values or outliers. This automation extends to the analysis itself, with AI models capable of sifting through vast amounts of data to identify trends, correlations, and insights that might be invisible or time-consuming for human analysts to uncover.
Natural Language Processing
Natural Language Processing (NLP), a subset of AI, automates the extraction of meaningful information from text data. This is particularly valuable in today’s data-rich environment where unstructured data abound, such as customer reviews, social media posts, and open-ended survey responses. NLP algorithms can analyze this text to extract sentiments, trends, and patterns, providing a deeper understanding of customer behavior and preferences.
Predicting Future Trends
Predictive analytics is another area where AI significantly enhances data analytics capabilities. By leveraging historical data, AI models can forecast future trends, behaviors, and outcomes with a high degree of accuracy.
Predictive Modeling
AI-driven predictive models use historical data to identify patterns and predict future events. This could mean forecasting sales trends, identifying potential market shifts, or predicting customer churn in business. These predictions allow organizations to make proactive decisions, such as adjusting inventory levels, tailoring marketing strategies, or developing new products to meet anticipated demand.
Time Series Analysis
Time series analysis, powered by AI, is crucial for understanding temporal patterns in data. AI models can analyze sequences of data points (time series) to forecast future values. This is invaluable in financial analysis for predicting stock prices, economic forecasting, and demand planning in supply chain management.
Enhancing Decision-Making
AI not only automates and predicts but also significantly enhances the decision-making process. By providing deeper insights and forecasts, AI-driven analytics empower decision-makers with actionable intelligence.
Decision Intelligence
Decision intelligence is an emerging field that combines data analytics with AI to improve decision-making. It involves modeling each decision as a set of processes, using AI to simulate outcomes and optimize decisions. This approach allows organizations to assess the potential impacts of their decisions before implementing them, minimizing risks and maximizing outcomes.
Augmented Analytics
Augmented analytics uses AI to enhance data analytics processes, making them more accessible to non-experts and expanding the scope of insights generated. AI algorithms can suggest areas of interest for further analysis, recommend visualizations that best communicate the insights, and generate natural language summaries of the data findings. This democratization of data analytics enables a broader range of stakeholders to participate in data-driven decision-making.
Best Practices for Integrating AI in Data Analytics
To effectively leverage AI in data analytics, organizations should consider the following best practices:
- Quality Data: AI models are only as good as the data they are trained on. Ensuring data quality through effective data governance and management practices is paramount.
- Ethical AI Use: Ethical considerations must be paramount as organizations rely more on AI for decisions. This includes ensuring privacy, fairness, and transparency in AI models’ development and use.
- Continuous Learning: AI models can drift over time as data and contexts change. Implementing continuous learning and model updating mechanisms is crucial for maintaining accuracy and relevance.
- Cross-disciplinary Teams: Effective AI integration requires collaboration between data scientists, domain experts, and decision-makers. Building cross-disciplinary teams ensures that AI solutions are technically sound and aligned with business objectives.
- Embrace Experimentation: The field of AI is rapidly evolving. Organizations should foster a culture of experimentation and learning to explore new AI applications and technologies.
Conclusion
Integrating AI into data analytics marks a significant leap forward in how organizations approach data-driven decision-making. AI streamlines analytics and provides deeper insights and foresight by automating analytical processes, predicting future trends, and enhancing decision-making. As businesses continue to navigate an increasingly data-rich environment, the role of AI in data analytics will only grow in importance, driving innovation, efficiency, and competitive advantage. The future of data analytics is not just about analyzing what has happened but about anticipating what will happen next, and AI is the key to unlocking that future.