AI Data Quality Matters—Bad Data Leads to AI Failure
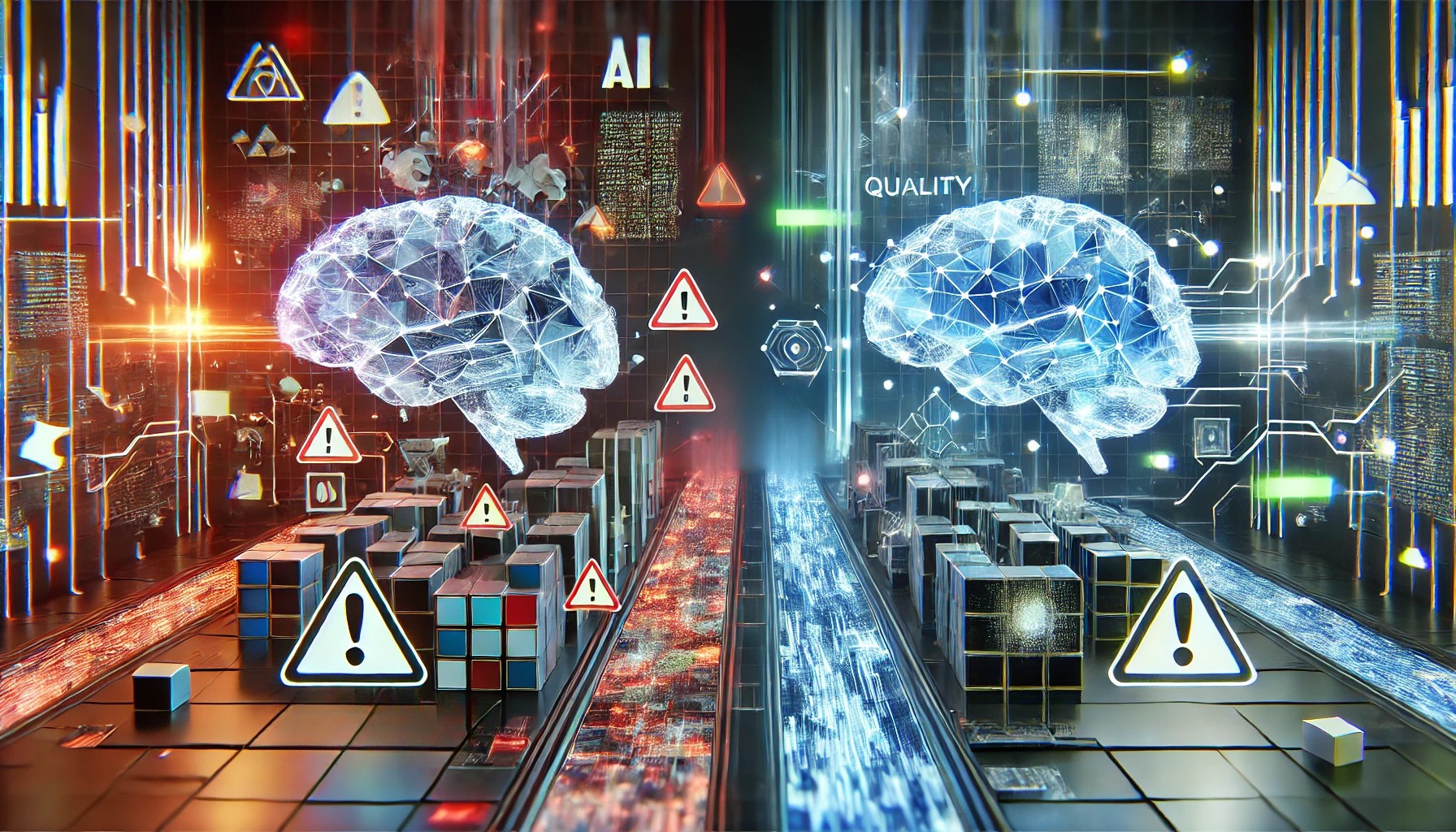
The Harsh Reality of AI
AI doesn’t fix bad data. It weaponizes it.
If your data is a mess, AI will amplify the chaos. Flawed inputs lead to flawed outputs. AI isn’t a savior—it’s an accelerant. Expect misleading insights, biased decisions, and operational breakdowns if your data isn’t clean, structured, and governed.
Businesses that treat AI as a silver bullet will find themselves dealing with bigger problems than they started with.
The Illusion of AI as a Fix-All
Many organizations rush into AI initiatives with high expectations. They assume AI will correct their data inconsistencies and automate decision-making seamlessly.
Reality check: AI isn’t magic. It’s a mirror. It reflects the quality of your data and processes—good or bad.
If you feed it garbage, it doesn’t just fail—it fails at scale. And when AI systems make bad decisions, they do so faster and more efficiently than humans ever could. That’s how minor data issues turn into full-blown operational crises.
The Silent Threat: AI Exposes Data Quality Gaps
AI reveals weaknesses you didn’t even know you had.
Many organizations operate under the assumption that their data is “good enough.” AI quickly proves otherwise. When AI processes incomplete, duplicated, or outdated data, the results aren’t just inaccurate—they’re dangerously misleading.
- Inconsistent data? AI learns the wrong patterns and produces unreliable predictions. Sales forecasts, risk models, and customer insights become distorted, leading to costly business decisions.
- Siloed data? AI lacks the full picture. Fragmented data across departments means AI only works with partial information, making recommendations that contradict real-world business realities.
- Poor governance? AI compliance risks skyrocket. Without clear data lineage and accountability, AI-driven decisions become black boxes—impossible to audit and prone to regulatory scrutiny.
- Data duplication and redundancy? AI will process the same information multiple times, leading to conflicting outputs and wasted resources.
- Bias and ethical concerns? If historical data is flawed or contains biases, AI will reinforce them, making discrimination and misinformation a real threat.
The cost of these gaps is significant. AI doesn’t just expose bad data—it amplifies its consequences. A miscalculated risk assessment, an incorrect pricing recommendation, or a biased hiring model can create financial, operational, and reputational disasters.
Why AI Demands a Strong Data Foundation
AI doesn’t need more data. It needs better data.
Data hoarding has been a corporate habit for decades. But AI doesn’t reward volume—it rewards quality. Organizations that dump all their data into AI models without governance, structure, or validation create massive liabilities.
- Data governance isn’t optional—it’s an AI prerequisite. Every AI model needs clear rules on what data it can use, where that data originates, and how it’s maintained over time. Without governance, AI decisions are unpredictable and untrustworthy.
- AI initiatives must start with a data strategy, not an algorithm. Many organizations invest in AI first, assuming they can figure out data issues later. This is backward. AI success depends on structured, consistent, and high-integrity data from day one.
- Scalability depends on data consistency, security, and accessibility. AI cannot operate efficiently if it’s constantly being fed fragmented, duplicate, or insecure data. Enterprise-wide data architecture must support AI with standardization and real-time accessibility.
- Regulatory compliance requires robust data management. AI-driven decisions are subject to increasing scrutiny. Companies that don’t invest in data governance risk non-compliance with regulations like GDPR, CCPA, and industry-specific mandates.
AI-ready organizations recognize that data maturity isn’t a one-time effort. It’s an ongoing discipline requiring investment in processes, technology, and talent. Without this foundation, AI remains a costly experiment with little business impact.
The Fix: Build AI-Ready Data, Not Hype
Most AI failures aren’t caused by bad models. They’re caused by bad data. Fix the foundation, and AI will deliver real business value. Here’s how:
- Clean your data before AI gets involved. AI thrives on accuracy, not volume. Organizations must establish rigorous data cleaning and validation protocols before deploying AI models. This includes removing duplicate records, standardizing data formats, and eliminating outdated or incomplete datasets.
- Break down silos with enterprise-wide data integration. AI requires seamless access to cross-functional data. Investing in data lakes, cloud-based architectures, and API-driven integrations ensures AI models can analyze complete datasets instead of isolated fragments.
- Implement governance before AI scales the problem. Data governance isn’t just about security—it’s about ensuring AI operates within controlled, compliant, and transparent frameworks. Organizations must define data ownership, access controls, and compliance rules before AI enters production.
- Prioritize explainability and accountability. AI decisions must be traceable. Business leaders need to understand why AI makes certain recommendations and have mechanisms in place to challenge or override faulty insights.
- Create an AI data strategy roadmap. Map out a phased approach to data readiness, ensuring AI aligns with business objectives and delivers measurable outcomes.
- Invest in upskilling and AI literacy. AI success isn’t just a technology issue—it’s a people issue. Equip teams with the skills to manage and interpret AI-driven insights effectively.
The Takeaway: AI Is Only as Smart as Your Data
AI doesn’t create competitive advantage—data quality does.
The organizations winning with AI aren’t chasing the latest models. They’re fixing their data problems before AI can exploit them. Those who neglect this reality will be left scrambling to patch issues after AI makes them impossible to ignore.
AI success starts long before implementation. It starts with a structured, disciplined approach to data management. Companies that invest in their data foundation today will be the AI leaders of tomorrow. Those who don’t will be left behind.
Fix your data now, or AI will expose the cracks for you.
The choice is yours.