Preparing Your Tech Stack for Generative AI: Integration Challenges and Solutions
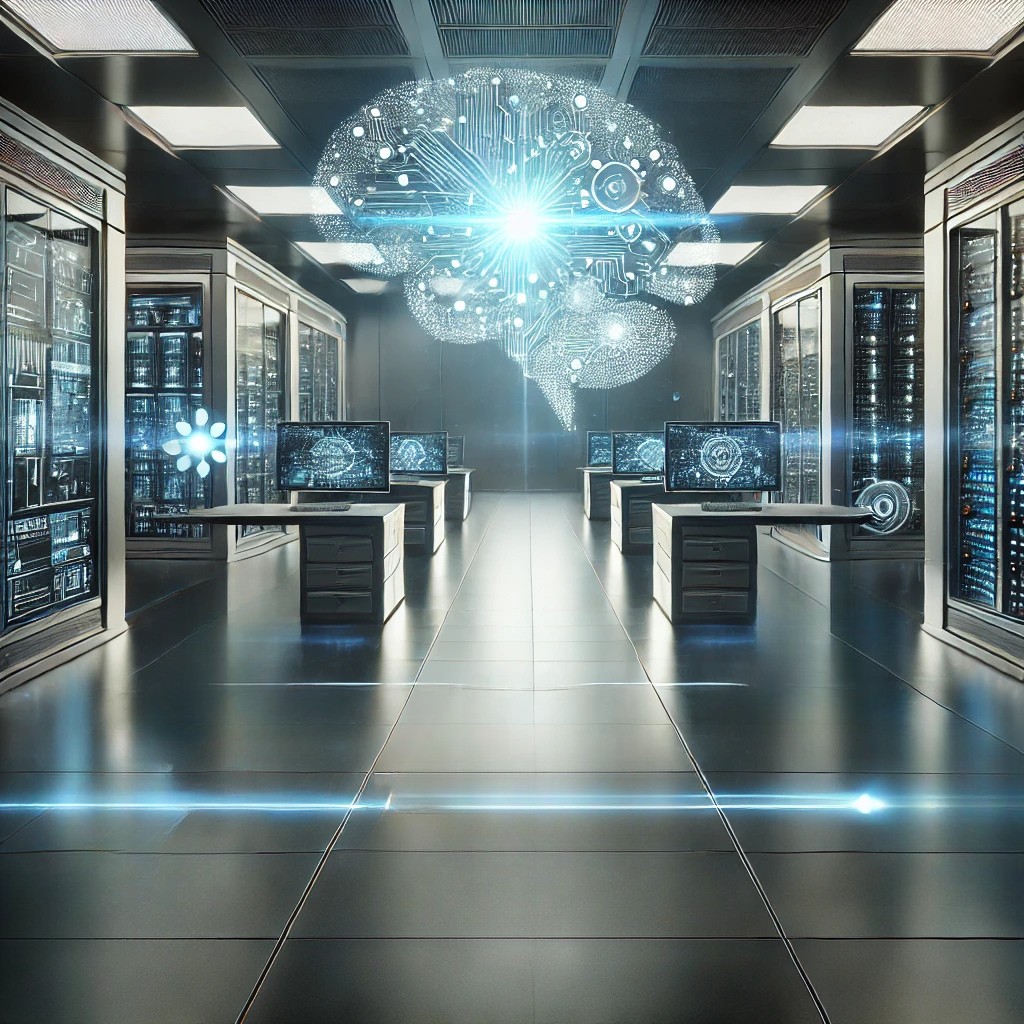
Generative AI has emerged as a game-changing technology, offering enterprises the ability to transform operations, create innovative solutions, and drive unparalleled efficiencies. But as with any cutting-edge innovation, its success relies heavily on the readiness of your organization’s technology infrastructure. A well-prepared tech stack is the foundation for seamless integration and impactful deployment. Here, we explore the key steps to assess and adapt your infrastructure, tackle integration challenges, and position your organization for long-term success with generative AI.
1. Assessing Your Current Infrastructure
Before implementing generative AI initiatives, it is crucial to evaluate the readiness of your existing tech stack. This process helps identify gaps and areas requiring upgrades or enhancements. Key areas to consider include:
- Data Readiness: Generative AI models rely on high-quality, well-organized data. Do you have access to clean and structured datasets that can fuel AI-driven insights?
- Compute Power: The computational demands of generative AI are significant, particularly for model training and real-time applications. Does your infrastructure include GPUs, TPUs, or other high-performance computing resources?
- Scalability: Generative AI projects often grow rapidly. Can your infrastructure scale to handle increased workloads as adoption expands?
- Integration Capabilities: How seamlessly can generative AI tools integrate with your existing systems, such as CRM, ERP, or other enterprise platforms?
2. Common Integration Challenges
Despite its potential, integrating generative AI into enterprise systems comes with several hurdles. CIOs and CTOs must anticipate and address these challenges:
- Legacy Systems: Older technologies often lack compatibility with modern AI platforms. Integration may require custom APIs, middleware, or a complete system overhaul.
- Data Silos: Fragmented data across departments limits AI’s ability to generate comprehensive insights. Breaking down these silos is essential for successful deployment.
- Security Risks: Integrating AI into sensitive enterprise systems raises concerns about data privacy and security. Ensuring robust governance and compliance frameworks is critical.
- Latency Issues: Many generative AI applications, such as chatbots and dynamic content generation, require low-latency environments, which can strain outdated networks.
3. Solutions for Overcoming Integration Challenges
Adopting generative AI successfully requires strategic investments and thoughtful planning. Here are practical solutions to overcome common integration challenges:
- Adopt Cloud-Native Platforms: Transitioning to cloud-based solutions offers the flexibility, scalability, and computational power required for generative AI. Platforms like AWS Sagemaker, Azure AI, and Google Vertex AI provide tailored tools for AI development and deployment.
- Invest in Data Management Systems: Implementing data lakes or data fabrics helps centralize and standardize data, making it more accessible and usable for AI models.
- Upgrade Networking and Storage: Ensure your infrastructure supports high-speed data transfers and storage capacity to handle AI’s intensive demands.
- Leverage Containerization: Tools like Kubernetes and Docker enable scalable, portable AI deployments, simplifying integration with existing systems.
- Prioritize Cybersecurity: Establish strong encryption, monitoring, and AI governance protocols to protect sensitive data and ensure compliance with regulations.
4. The Role of Cross-Functional Collaboration
Integrating generative AI isn’t just a technical endeavor—it requires alignment across multiple teams. Collaboration ensures that all aspects of the initiative, from data preparation to business impact, are addressed:
- IT Teams: Oversee the technical aspects of integration and infrastructure upgrades.
- Data Teams: Ensure data is clean, structured, and ready for AI training.
- Business Leaders: Align AI projects with strategic goals to prioritize use cases that deliver measurable ROI.
5. Building a Long-Term Vision
Preparing your tech stack for generative AI is not a one-time effort but an ongoing journey. To future-proof your infrastructure:
- Invest in AI-Optimized Hardware: High-performance GPUs, TPUs, and edge computing devices are essential for efficient AI processing.
- Implement MLOps Practices: Establish workflows for continuous development, deployment, and monitoring of AI models to ensure they remain effective over time.
- Monitor Emerging Technologies: Stay informed about advancements in AI platforms, data management tools, and integration solutions to maintain a competitive edge.
Conclusion
Generative AI represents a transformative opportunity for enterprises, but its potential can only be unlocked with the right technological foundation. By assessing your current infrastructure, addressing integration challenges, and investing in scalable solutions, CIOs and CTOs can position their organizations to fully harness the power of generative AI. Now is the time to future-proof your tech stack and lead your organization into the next era of innovation.
Ready to take the next step? Contact Data Ideology to explore how we can help you prepare your infrastructure for generative AI success.